In the ever-changing field of artificial intelligence (AI), 2024 promises substantial advances with the introduction of generative AI, particularly through a cutting-edge technique known as retrieval-augmented generation (RAG). This novel approach combines large language models (LLMs) and domain-specific databases to improve their capacity to deliver contextually appropriate responses. The consequences are enormous, with the potential to revolutionize how businesses use AI to increase production and efficiency.
Understanding RAG: Bridging AI with Domain-Specific Knowledge
At its core, retrieval-augmented generation (RAG) combines powerful AI capabilities with curated domain knowledge. By connecting LLMs to specialized databases containing massive amounts of information ranging from technical documentation to scientific literature and financial filings, RAG enables AI systems to produce more precise and contextually relevant results. This is accomplished using a dual-process technique in which the model first gets relevant information from the database before generating replies based on that data.
Challenges and Innovations: Benchmarking RAG Systems
Despite its potential, RAG implementation presents problems. One of the biggest challenges is assessing the efficacy of various RAG methods across multiple domains. To address this, researchers at Amazon Web Services (AWS) have developed a revolutionary solution based on automated benchmarking. This method provides customized multiple-choice exams that evaluate how well RAG systems answer domain-specific questions. Such assessments not only standardize testing techniques, but also ensure the scalability and interpretability of RAG deployments.
Key Findings: Optimizing RAG for Enhanced AI Performance
Recent research provides critical insights toward enhancing RAG systems. Contrary to popular opinion, scaling up LLM size does not ensure improved performance; instead, the retrieval method chosen is critical. Researchers discovered that using the correct retrieval strategy can dramatically improve AI skills, sometimes beating benefits made only by increasing model size. This nuanced approach not only improves performance but also resolves concerns about the resource requirements of large-scale AI implementations.
Practical Implications: Deploying RAG in Enterprise Settings
For businesses navigating the difficulties of AI integration, RAG provides a strategic advantage. Businesses can improve client interactions, decision-making processes, and operations by successfully exploiting domain-specific knowledge. Applications range from customer service automation and technical support to financial analysis and scientific research.
Future Directions: Advancing RAG and Beyond
Looking ahead, RAG’s growth has the potential to significantly revolutionize the AI field. Continued research and development into optimizing retrieval methods, improving algorithmic accuracy, and expanding database integration will be vital. These breakthroughs promise to open up new possibilities for enterprise AI, opening the path for smarter, more efficient solutions to address the changing needs of modern enterprises.
As organizations recognize the potential of generative AI and retrieval-augmented generation, the journey to effectively harnessing these technologies begins. Understanding the subtleties of RAG, carefully assessing its effectiveness, and optimizing its implementation allows businesses to use AI to promote innovation, productivity, and growth in the digital world. The future of enterprise AI seems promising, with RAG positioned to play a transformative role in designing tomorrow’s intelligent systems.
Related Stories:
- AUDI’S VOICE CONTROL REVOLUTION: BOOSTED BY CHATGPT FOR SMARTER DRIVING
- WHY GOOGLE COLAB IS SO POPULAR: KEY BENEFITS AND REASONS
- APPLE ’S NEW STRATEGY: A SHIFT TOWARD AI AND SOFTWARE
Advertisement
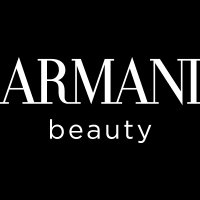
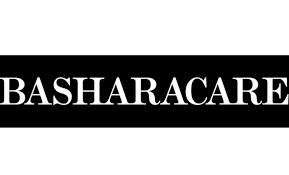
c
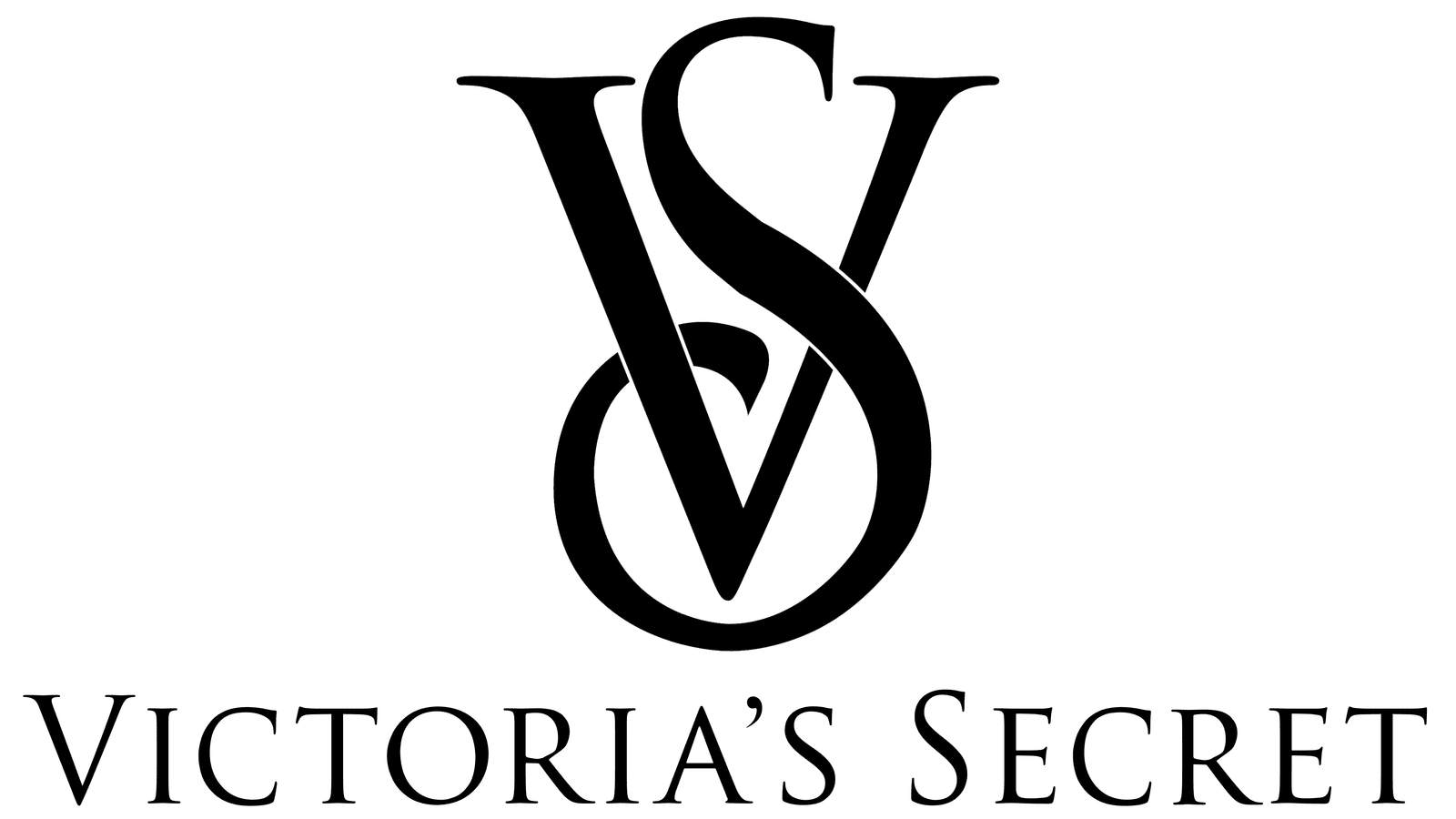