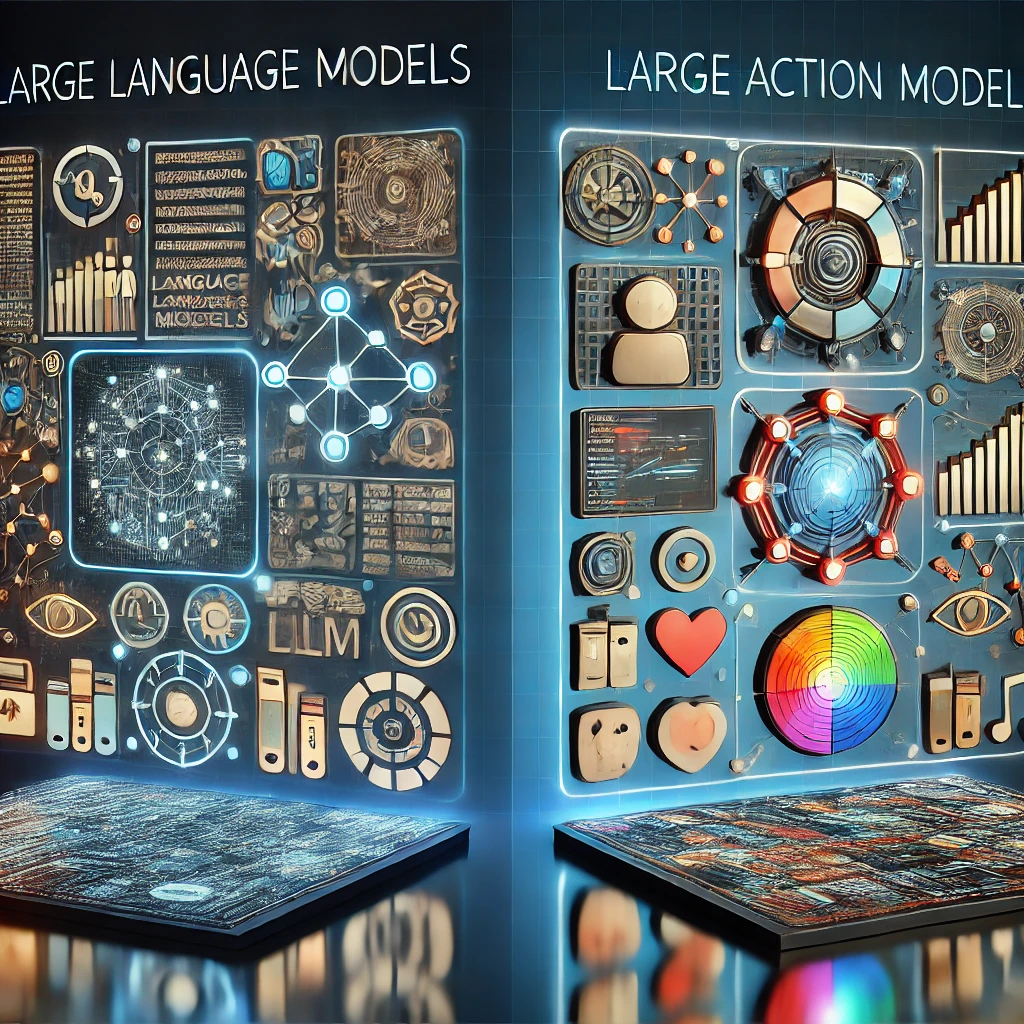
In the fast evolving field of artificial intelligence, two strong models stand out as milestones in our path to artificial general intelligence: Large Language Models (LLMs) and Large Action Models. While LLMs have paved the way for language interpretation and creation, LAMs have expanded AI’s capabilities beyond words to include numerous data kinds, resulting in a multimodal AI experience. Let’s look at the fascinating transition from LLMs to LAMs and how they’re transforming AI.
Coupons for better shopping on brands
The Basics: LLMs vs. LAMs
Large Language Models (LLMs) are primarily intended to interpret, analyze, and create text. Examples include OpenAI’s GPT series and Google’s BERT, which have allowed applications in language translation, customer assistance, and content creation. However, LLMs are confined to text-based data, which means they excel at language tasks but are unable to read other data sources.
Large Action Models (LAMs) are the next generation, designed to comprehend and act on a variety of data kinds, or modalities. This means that LAMs can process not only text, but also pictures, audio, and maybe video or sensor data. LAMs are meant to easily integrate and interpret many types of input, bringing us closer to an AI that can perceive and respond to the world.
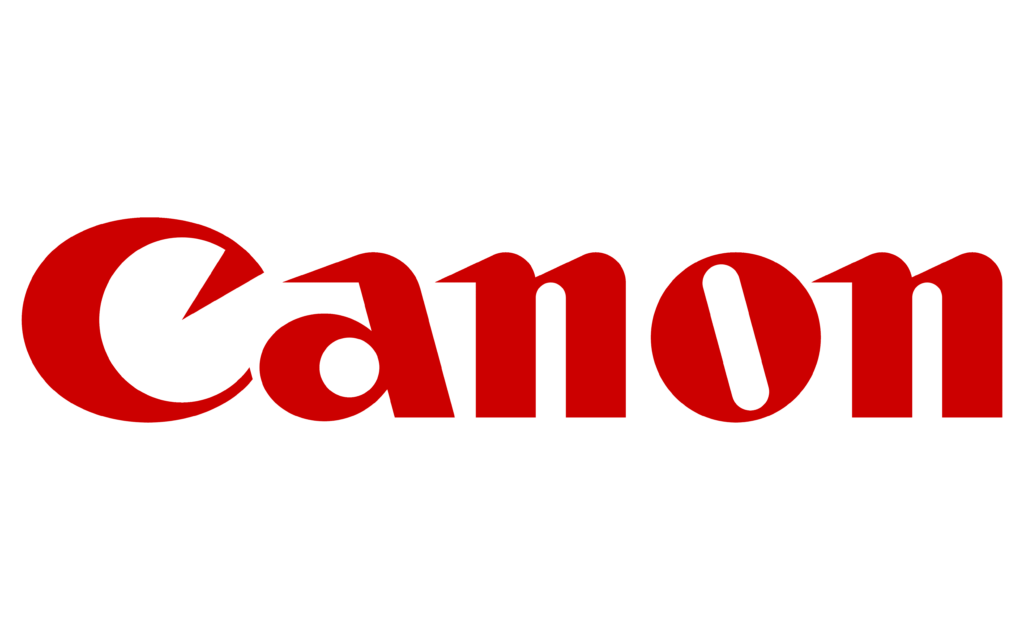
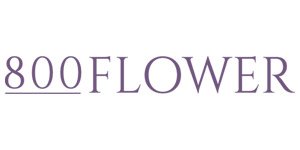
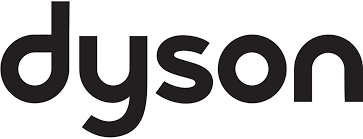
Dyson
Key Differences
Data Modalities
LLMs: These models are adept at processing and creating text, and while they add sophistication to language-based applications, they struggle with non-text input.
LAMs: Can comprehend and respond on multimodal data inputs, providing a larger range of knowledge. They can analyze a variety of text, audio, and visual data, making them useful in sectors where numerous data types exist.
Process and Interpretation
LLMs are designed to be unimodal, with an emphasis on text processing, coherent response generation, and language understanding.
In contrast, the nature of the LAM is intrinsically multimodal, it may cover information from a variety of different data types. Taking video conversations as just one example, the input data for LAMs would be both verbal and visual in nature. It is such an aspect that provides situational awareness, impossible within the scope of LLMs.
Action-Oriented Design
LLMs are mostly text-based responders, with activities confined to creating or analyzing text-based data.
LAMs: These models are designed to act on the information they collect from many data sources. They might hypothetically detect objects in photos, make judgements based on aural cues, and respond to precise visual or contextual suggestions.
For exciting offers on brands click here
Complexity and Real-World Applications
LLMs excel in language-based tasks, making them ideal for applications such as content production, customer service, and text analytics.
LAMs: Designed for increasingly complicated, context-aware tasks that may include the synthesis of textual, visual, and aural input, making them useful in fields such as autonomous systems, multimedia analysis, and virtual reality.
Training Approaches
LLMs are trained on large text corpora, frequently utilizing unsupervised learning techniques to predict language patterns and semantics.
LAMs are trained on multimodal datasets, often using supervised or complicated reinforcement learning techniques, to successfully manage and integrate different data forms.
Why Are LAMs So Important?
LAMs are crucial in the field of artificial general intelligence (AGI) as they integrate various data types, mirroring human perception. They offer holistic perception, dynamic decision-making, and enhanced user experiences. By analyzing visual cues alongside spoken or written language, LAMs can make recommendations and interact with their environment, making AI more adaptable and responsive.
Current State and Future Prospects for LAMs
LAMs, while still experimental, have potential applications in healthcare, customer service, entertainment, and education. They can analyze patient speech, facial expressions, and clinical data in real-time, provide accurate diagnostics, adapt responses based on language and emotional cues, Multimodal AI has the potential to revolutionize entertainment by allowing AI characters to respond dynamically to player actions in video games or interactive media and provide personalized, immersive learning experiences. This model represent a significant advancement towards AGI, enabling AI systems to respond more context-awarely, paving the way for machines that can interact with humans and the environment effectively.
LLMs transition to LAMs, a multimodal approach, integrating multiple data types for a more responsive AI experience. Despite being emerging, their impact on AI and society is profound, moving closer to artificial general intelligence.
* This article contains affiliate links; if you click such a link and make a purchase, Doer Digitalz FZE may earn a commission